Convert a PaddlePaddle Model to ONNX and OpenVINO IR¶
This notebook shows how to convert a MobileNetV3 model from PaddleHub, pretrained on the ImageNet dataset, to OpenVINO IR. It also shows how to perform classification inference on a sample image using OpenVINO’s Inference Engine and compares the results of the PaddlePaddle model with the IR model.
Source of the model.
Preparation¶
Imports¶
import os
import time
import cv2
import matplotlib.pyplot as plt
import numpy as np
import paddlehub as hub
from IPython.display import Markdown, display
from PIL import Image
from openvino.runtime import Core
from paddle.static import InputSpec
from scipy.special import softmax
/opt/hostedtoolcache/Python/3.8.12/x64/lib/python3.8/site-packages/paddle/vision/transforms/functional_pil.py:36: DeprecationWarning: NEAREST is deprecated and will be removed in Pillow 10 (2023-07-01). Use Resampling.NEAREST or Dither.NONE instead.
'nearest': Image.NEAREST,
/opt/hostedtoolcache/Python/3.8.12/x64/lib/python3.8/site-packages/paddle/vision/transforms/functional_pil.py:37: DeprecationWarning: BILINEAR is deprecated and will be removed in Pillow 10 (2023-07-01). Use Resampling.BILINEAR instead.
'bilinear': Image.BILINEAR,
/opt/hostedtoolcache/Python/3.8.12/x64/lib/python3.8/site-packages/paddle/vision/transforms/functional_pil.py:38: DeprecationWarning: BICUBIC is deprecated and will be removed in Pillow 10 (2023-07-01). Use Resampling.BICUBIC instead.
'bicubic': Image.BICUBIC,
/opt/hostedtoolcache/Python/3.8.12/x64/lib/python3.8/site-packages/paddle/vision/transforms/functional_pil.py:39: DeprecationWarning: BOX is deprecated and will be removed in Pillow 10 (2023-07-01). Use Resampling.BOX instead.
'box': Image.BOX,
/opt/hostedtoolcache/Python/3.8.12/x64/lib/python3.8/site-packages/paddle/vision/transforms/functional_pil.py:40: DeprecationWarning: LANCZOS is deprecated and will be removed in Pillow 10 (2023-07-01). Use Resampling.LANCZOS instead.
'lanczos': Image.LANCZOS,
/opt/hostedtoolcache/Python/3.8.12/x64/lib/python3.8/site-packages/paddle/vision/transforms/functional_pil.py:41: DeprecationWarning: HAMMING is deprecated and will be removed in Pillow 10 (2023-07-01). Use Resampling.HAMMING instead.
'hamming': Image.HAMMING
Settings¶
Set IMAGE_FILENAME
to the filename of an image to use. Set
MODEL_NAME
to the PaddlePaddle model to download from PaddleHub.
MODEL_NAME
will also be the base name for the converted ONNX and IR
models. The notebook is tested with the
mobilenet_v3_large_imagenet_ssld
model. Other models may use different preprocessing methods and
therefore require some modification to get the same results on the
original and converted model.
hub.config.server
is the URL to the PaddleHub server. You should not
need to modify this setting.
IMAGE_FILENAME = "coco_close.png"
MODEL_NAME = "mobilenet_v3_large_imagenet_ssld"
hub.config.server = "https://paddlepaddle.org.cn/paddlehub"
Show Inference on PaddlePaddle Model¶
In the next cell, we load and download the model from PaddleHub, read and display an image, do inference on that image, and show the top three prediction results.
The first time you run this notebook, the PaddlePaddle model is downloaded from PaddleHub. This may take a while.
classifier = hub.Module(name=MODEL_NAME)
# Load image in BGR format, as specified in model documentation
image = cv2.imread(filename=IMAGE_FILENAME)
plt.imshow(cv2.cvtColor(image, cv2.COLOR_BGR2RGB))
result = classifier.classification(images=[image], top_k=3)
for class_name, softmax_probability in result[0].items():
print(f"{class_name}, {softmax_probability:.5f}")
[33m[2022-05-31 15:32:45,778] [ WARNING][0m - The _initialize method in HubModule will soon be deprecated, you can use the __init__() to handle the initialization of the object[0m
Labrador retriever, 0.58936
flat-coated retriever, 0.03327
curly-coated retriever, 0.03317
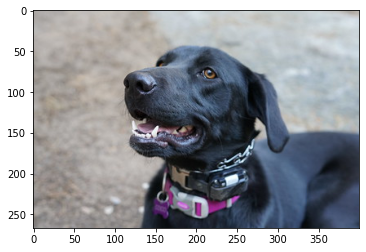
classifier.classification()
takes an image as input and returns the
class name of the image. By default the best network result is returned.
With the top_k
parameter, the best k
results are returned, where
k
is a number. Preprocessing the image and converting the network
result to class names is done behind the scenes. The classification
model returns an array with floating point values for each of the 1000
ImageNet classes. The higher the value, the more confident the network
is that the class number corresponding to that value (the index of that
value in the network output array) is the class number for the image.
The classification()
function converts these numbers to class names
and softmax
probabilities.
To see PaddlePaddle’s implementation for the classification function and for loading and preprocessing data, uncomment the next two cells.
# classifier??
# import mobilenet_v3_large_imagenet_ssld.data_feed
# %load $mobilenet_v3_large_imagenet_ssld.data_feed.__file__
The data_feed
module shows that images are normalized, resized and
cropped and that the BGR image is converted to RGB before propagating
through the network. In the next cell, we import the process_image
function that is defined in this file to do inference on the OpenVINO IR
model with the same method.
from mobilenet_v3_large_imagenet_ssld.data_feed import process_image
It is useful to show the output of the process_image()
function, to
see the effect of cropping and resizing. Because of the normalization,
the colors will look strange, and matplotlib will warn about clipping
values.
pil_image = Image.open(IMAGE_FILENAME)
processed_image = process_image(pil_image)
print(f"Processed image shape: {processed_image.shape}")
# Processed image is in (C,H,W) format, convert to (H,W,C) to show the image
plt.imshow(np.transpose(processed_image, (1, 2, 0)))
Processed image shape: (3, 224, 224)
Clipping input data to the valid range for imshow with RGB data ([0..1] for floats or [0..255] for integers).
<matplotlib.image.AxesImage at 0x7fe8d8b07430>
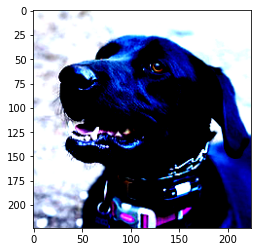
Convert the Model to OpenVINO IR Format¶
To Convert the PaddlePaddle model to IR, we first convert the model to ONNX, and then convert the ONNX model to IR.
Preparation¶
PaddlePaddle’s MobileNet model contains information about the input shape, mean and scale values that we can use to convert the model. The next cell shows how to get these values.
input_shape = list(classifier.cpu_predictor.get_input_tensor_shape().values())
print("input shape:", input_shape)
print("mean:", classifier.get_pretrained_images_mean())
print("std:", classifier.get_pretrained_images_std())
input shape: [[-1, 3, 224, 224]]
mean: [[0.485 0.456 0.406]]
std: [[0.229 0.224 0.225]]
Convert PaddlePaddle Model to ONNX¶
We convert the PaddlePaddle Model to ONNX with the
.export_onnx_model()
method. This uses
Paddle2ONNX. We
convert the model with the input shape found in the previous cell.
target_height, target_width = next(iter(input_shape))[2:]
x_spec = InputSpec([1, 3, target_height, target_width], "float32", "x")
print(
"Exporting PaddlePaddle model to ONNX with target_height "
f"{target_height} and target_width {target_width}"
)
classifier.export_onnx_model(".", input_spec=[x_spec], opset_version=11)
Exporting PaddlePaddle model to ONNX with target_height 224 and target_width 224
2022-05-31 15:32:48 [INFO] ONNX model saved in ./mobilenet_v3_large_imagenet_ssld.onnx
Convert ONNX model to OpenVINO IR Format¶
Call the OpenVINO Model Optimizer tool to convert the PaddlePaddle model
to OpenVINO IR, with FP32 precision. The models are saved to the current
directory. We can add the mean values to the model with
--mean_values
and scale the output with the standard deviation with
--scale_values
. With these options, it is not necessary to normalize
input data before propagating it through the network. However, to get
the exact same output as the PaddlePaddle model, it is necessary to
preprocess in the image in the same way. For this tutorial, we therefore
do not add the mean and scale values to the model, and we use the
process_image
function, as described in the previous section, to
ensure that both the IR and the PaddlePaddle model use the same
preprocessing methods. We do show how to get the mean and scale values
of the PaddleGAN model, so you can add them to the Model Optimizer
command if you want. See the PyTorch/ONNX to
OpenVINO
notebook for a notebook where these options are used.
Run ! mo --help
in a code cell to show an overview of command line
options for Model Optimizer. See the Model Optimizer Developer
Guide
for more information about Model Optimizer.
In the next cell, we first construct the command for Model Optimizer,
and then execute this command in the notebook by prepending the command
with a !
. Model Optimization was succesful if the last lines of the
output include [ SUCCESS ] Generated IR version 11 model
.
model_xml = f"{MODEL_NAME}.xml"
if not os.path.exists(model_xml):
mo_command = f'mo --input_model {MODEL_NAME}.onnx --input_shape "[1,3,{target_height},{target_width}]"'
display(Markdown(f"Model Optimizer command to convert the ONNX model to IR: `{mo_command}`"))
display(Markdown("_Converting model to IR. This may take a few minutes..._"))
! $mo_command
else:
print(f"{model_xml} already exists.")
Model Optimizer command to convert the ONNX model to IR:
mo --input_model mobilenet_v3_large_imagenet_ssld.onnx --input_shape "[1,3,224,224]"
Converting model to IR. This may take a few minutes…
Model Optimizer arguments:
Common parameters:
- Path to the Input Model: /home/runner/work/openvino_notebooks/openvino_notebooks/notebooks/103-paddle-onnx-to-openvino/mobilenet_v3_large_imagenet_ssld.onnx
- Path for generated IR: /home/runner/work/openvino_notebooks/openvino_notebooks/notebooks/103-paddle-onnx-to-openvino/.
- IR output name: mobilenet_v3_large_imagenet_ssld
- Log level: ERROR
- Batch: Not specified, inherited from the model
- Input layers: Not specified, inherited from the model
- Output layers: Not specified, inherited from the model
- Input shapes: [1,3,224,224]
- Source layout: Not specified
- Target layout: Not specified
- Layout: Not specified
- Mean values: Not specified
- Scale values: Not specified
- Scale factor: Not specified
- Precision of IR: FP32
- Enable fusing: True
- User transformations: Not specified
- Reverse input channels: False
- Enable IR generation for fixed input shape: False
- Use the transformations config file: None
Advanced parameters:
- Force the usage of legacy Frontend of Model Optimizer for model conversion into IR: False
- Force the usage of new Frontend of Model Optimizer for model conversion into IR: False
OpenVINO runtime found in: /opt/hostedtoolcache/Python/3.8.12/x64/lib/python3.8/site-packages/openvino
OpenVINO runtime version: 2022.1.0-7019-cdb9bec7210-releases/2022/1
Model Optimizer version: 2022.1.0-7019-cdb9bec7210-releases/2022/1
[ SUCCESS ] Generated IR version 11 model.
[ SUCCESS ] XML file: /home/runner/work/openvino_notebooks/openvino_notebooks/notebooks/103-paddle-onnx-to-openvino/mobilenet_v3_large_imagenet_ssld.xml
[ SUCCESS ] BIN file: /home/runner/work/openvino_notebooks/openvino_notebooks/notebooks/103-paddle-onnx-to-openvino/mobilenet_v3_large_imagenet_ssld.bin
[ SUCCESS ] Total execution time: 0.76 seconds.
[ SUCCESS ] Memory consumed: 120 MB.
It's been a while, check for a new version of Intel(R) Distribution of OpenVINO(TM) toolkit here https://software.intel.com/content/www/us/en/develop/tools/openvino-toolkit/download.html?cid=other&source=prod&campid=ww_2022_bu_IOTG_OpenVINO-2022-1&content=upg_all&medium=organic or on the GitHub*
[ INFO ] The model was converted to IR v11, the latest model format that corresponds to the source DL framework input/output format. While IR v11 is backwards compatible with OpenVINO Inference Engine API v1.0, please use API v2.0 (as of 2022.1) to take advantage of the latest improvements in IR v11.
Find more information about API v2.0 and IR v11 at https://docs.openvino.ai
Show Inference on OpenVINO Model¶
Load the IR model, get model information, load the image, do inference, convert the inference to a meaningful result, and show the output. See the Inference Engine API Notebook for more information.
# Load Inference Engine and IR model
ie = Core()
model = ie.read_model(model=f"{MODEL_NAME}.xml", weights=f"{MODEL_NAME}.bin")
compiled_model = ie.compile_model(model=model, device_name="CPU")
# Get model output
output_layer = compiled_model.output(0)
# Read, show, and preprocess input image
# See the "Show Inference on PaddlePaddle Model" section for source of process_image
image = Image.open(IMAGE_FILENAME)
plt.imshow(image)
input_image = process_image(image)[None,]
# Do inference
ie_result = compiled_model([input_image])[output_layer][0]
# Compute softmax probabilities for the inference result and find the top three values
softmax_result = softmax(ie_result)
top_indices = np.argsort(softmax_result)[-3:][::-1]
top_softmax = softmax_result[top_indices]
# Convert the inference results to class names, using the same labels as the PaddlePaddle classifier
for index, softmax_probability in zip(top_indices, top_softmax):
print(f"{classifier.label_list[index]}, {softmax_probability:.5f}")
Labrador retriever, 0.58936
flat-coated retriever, 0.03327
curly-coated retriever, 0.03317
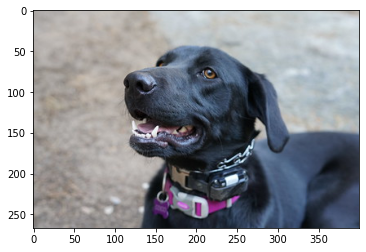
Timing and Comparison¶
Measure the time it takes to do inference on fifty images and compare the result. The timing information gives an indication of performance. For a fair comparison, we include the time it takes to process the image. For more accurate benchmarking, use the OpenVINO benchmark tool. Note that many optimizations are possible to improve the performance.
num_images = 50
# PaddlePaddle's classification method expects a BGR numpy array
image = cv2.imread(filename=IMAGE_FILENAME)
# The process_image function expects a PIL image
pil_image = Image.open(fp=IMAGE_FILENAME)
# Show CPU information
ie = Core()
print(f"CPU: {ie.get_property(device_name='CPU', name='FULL_DEVICE_NAME')}")
CPU: Intel(R) Xeon(R) Platinum 8272CL CPU @ 2.60GHz
# Show inference speed on PaddlePaddle model
start = time.perf_counter()
for _ in range(num_images):
result = classifier.classification(images=[image], top_k=3)
end = time.perf_counter()
time_ir = end - start
print(
f"PaddlePaddle model on CPU: {time_ir/num_images:.4f} "
f"seconds per image, FPS: {num_images/time_ir:.2f}\n"
)
print("PaddlePaddle result:")
for class_name, softmax_probability in result[0].items():
print(f"{class_name}, {softmax_probability:.5f}")
plt.imshow(cv2.cvtColor(image, cv2.COLOR_BGR2RGB));
PaddlePaddle model on CPU: 0.0437 seconds per image, FPS: 22.91
PaddlePaddle result:
Labrador retriever, 0.58936
flat-coated retriever, 0.03327
curly-coated retriever, 0.03317
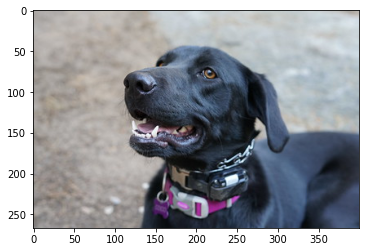
# Show inference speed on OpenVINO IR model
compiled_model = ie.compile_model(model=model, device_name="CPU")
output_layer = compiled_model.output(0)
start = time.perf_counter()
input_image = process_image(pil_image)[None,]
for _ in range(num_images):
ie_result = compiled_model([input_image])[output_layer][0]
result_index = np.argmax(ie_result)
class_name = classifier.label_list[np.argmax(ie_result)]
softmax_result = softmax(ie_result)
top_indices = np.argsort(softmax_result)[-3:][::-1]
top_softmax = softmax_result[top_indices]
end = time.perf_counter()
time_ir = end - start
print(
f"IR model in Inference Engine (CPU): {time_ir/num_images:.4f} "
f"seconds per image, FPS: {num_images/time_ir:.2f}"
)
print()
print("OpenVINO result:")
for index, softmax_probability in zip(top_indices, top_softmax):
print(f"{classifier.label_list[index]}, {softmax_probability:.5f}")
plt.imshow(cv2.cvtColor(image, cv2.COLOR_BGR2RGB));
IR model in Inference Engine (CPU): 0.0044 seconds per image, FPS: 229.56
OpenVINO result:
Labrador retriever, 0.58936
flat-coated retriever, 0.03327
curly-coated retriever, 0.03317
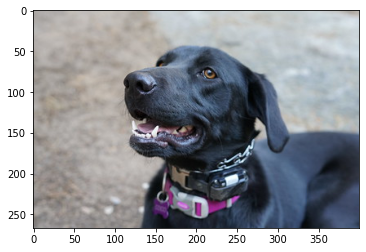